Wound detection is an essential aspect of wound assessment that enables healthcare professionals to determine the severity of tissue damage and provide the appropriate course of treatment. However, traditional wound depth detection methods can be prone to subjective measurements and errors. Fortunately, advancements in artificial intelligence (AI) and synthetic data generation have revolutionized the field of wound depth detection.
Traditional wound depth detection methods
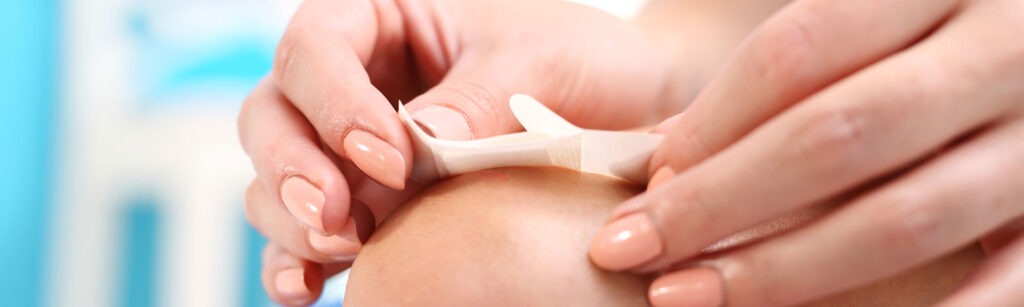
One of the main challenges of traditional wound depth detection methods is the subjectivity of the measurements. In some cases, healthcare professionals use visual inspection to estimate the depth of a wound, which can lead to measurement inconsistencies. Other methods, such as probes or ultrasound, can be invasive, time-consuming, and expensive.
Synthetic data generation
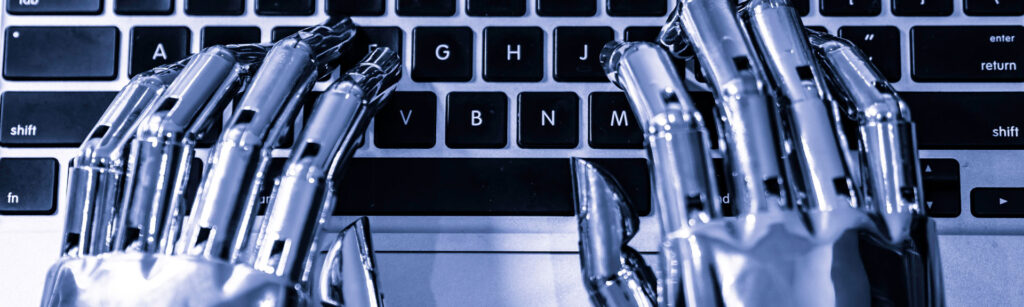
Researchers have turned to AI and synthetic data generation to overcome these challenges to develop more accurate and efficient wound depth detection methods. Synthetic data can mimic real-world scenarios, which can help overcome the challenges associated with real-world data. Different tools, such as SculptGL for creating a wound, Paint3D for improving generated synthetic data, and Online 3D Viewer for measuring the angled distance from skin to the deepest point of the wound and for measuring the horizontal length between the two points, are used.
Using synthetic data can significantly reduce the time, cost, and privacy concerns associated with data collection while allowing machine learning models to detect wound depth accurately. AI algorithms learn to detect wound depth by analyzing images of wounds and correlating wound features with the known depths of similar wounds. Synthetic data can simulate wounds of varying depths, shapes, and sizes, enabling machine-learning models to recognize patterns indicative of different levels of wound depth.
DDI Team, has developed an AI system using 3D synthetic data similar to real wound images. The system uses a convolutional neural network (CNN) to detect wound depth from images. The team trained the CNN using a dataset of 3D synthetic wound images generated with SculptGL and Paint3D. The synthetic images were designed to simulate wounds of varying depths, shapes, and sizes.
Using AI and synthetic data generation in wound depth detection has several benefits. First, it can reduce the subjectivity and errors associated with traditional wound depth detection methods. Second, it can save time and reduce costs related to data collection. Third, it can enable healthcare professionals to make more accurate and timely decisions regarding wound care, leading to better patient outcomes.
The use of AI and synthetic data generation has revolutionized the field of wound depth detection. The method allows for more accurate and efficient wound depth detection, which can lead to better patient outcomes. The development of the AI system by the DDI Team using synthetic data and machine learning is an excellent example of the potential of this technology in the healthcare field. As AI advances, we can expect to see more innovative solutions to healthcare challenges.